
Computers and Technology, 10.03.2020 19:36 kris7726
The average perceptron will add a modification to the original perceptron algorithm: since the basic algorithm continues updating as the algorithm runs, nudging parameters in possibly conflicting directions, it is better to take an average of those parameters as the final answer. Every update of the algorithm is the same as before. The returned parameters θ, however, are an average of the θs across the nT steps:
θfinal=1/nT(θ^(1)+θ^(2)+...+θ^(nT))
You will now implement the average perceptron algorithm. This function should be constructed similarly to the Full Perceptron Algorithm above, except that it should return the average values of θ and θ0
Tip: Tracking a moving average through loops is difficult, but tracking a sum through loops is simple.
Note: Please call get_order(feature_matrix. shape[0]), and use the ordering to iterate the feature matrix in each iteration. The ordering is specified due to grading purpose. In practice, people typically just randomly shuffle indices to do stochastic optimization.
Available Functions: You have access to the NumPy python library as np and perceptron_single_step_update which you have already implemented.
def average_perceptron(feature_matrix, labels, T):
"""
Runs the average perceptron algorithm on a given set of data. Runs T
iterations through the data set, there is no need to worry about
stopping early.
NOTE: Please use the previously implemented functions when applicable.
Do not copy paste code from previous parts.
NOTE: Iterate the data matrix by the orders returned by get_order(feature_matrix. shape[0])
Args:
feature_matrix - A numpy matrix describing the given data. Each row
represents a single data point.
labels - A numpy array where the kth element of the array is the
correct classification of the kth row of the feature matrix.
T - An integer indicating how many times the perceptron algorithm
should iterate through the feature matrix.
Returns: A tuple where the first element is a numpy array with the value of
the average theta, the linear classification parameter, found after T
iterations through the feature matrix and the second element is a real
number with the value of the average theta_0, the offset classification
parameter, found after T iterations through the feature matrix.
Hint: It is difficult to keep a running average; however, it is simple to
find a sum and divide.
"""
# Your code here
raise NotImplementedError

Answers: 1
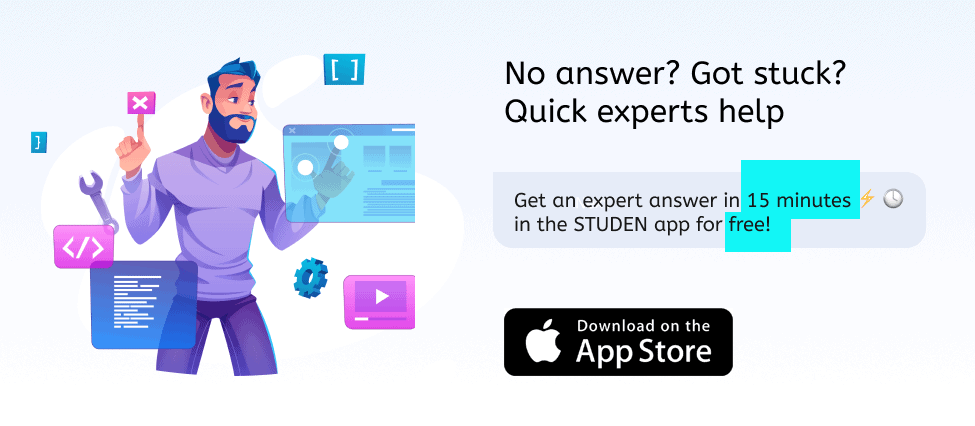
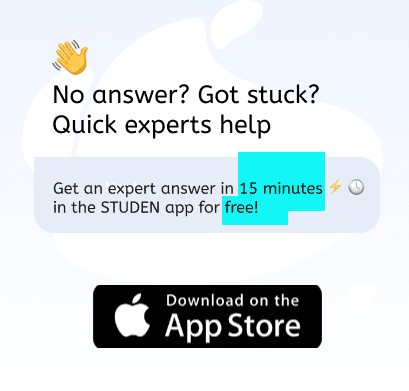
Other questions on the subject: Computers and Technology

Computers and Technology, 22.06.2019 04:50, edenlbarfield
Which are steps taken to diagnose a computer problem? a) reproducing the problem and using error codes b) reproducing the problem and troubleshooting c) using error codes and troubleshooting d) using error codes and stepping functions
Answers: 1

Computers and Technology, 22.06.2019 23:30, elizabethburkha
Which text format is this, "the text is transcribed exactly as it sounds and includes all the utterances of the speakers. "?
Answers: 2


Computers and Technology, 23.06.2019 14:00, savannnab1890
Select the correct answer. andre was recently hired by an organization to check for system vulnerabilities. he is supposed to exploit these vulnerabilities and create a report on the extent of damage to which the system was susceptible. what position does andre hold in this organization? a. information security analyst b. information assurance manager c. penetration tester d. network security engineer e. chief information security officer
Answers: 2
You know the right answer?
The average perceptron will add a modification to the original perceptron algorithm: since the basic...
Questions in other subjects:

History, 30.05.2021 05:00

Mathematics, 30.05.2021 05:00

Mathematics, 30.05.2021 05:00


Mathematics, 30.05.2021 05:00


Biology, 30.05.2021 05:00

Mathematics, 30.05.2021 05:00
